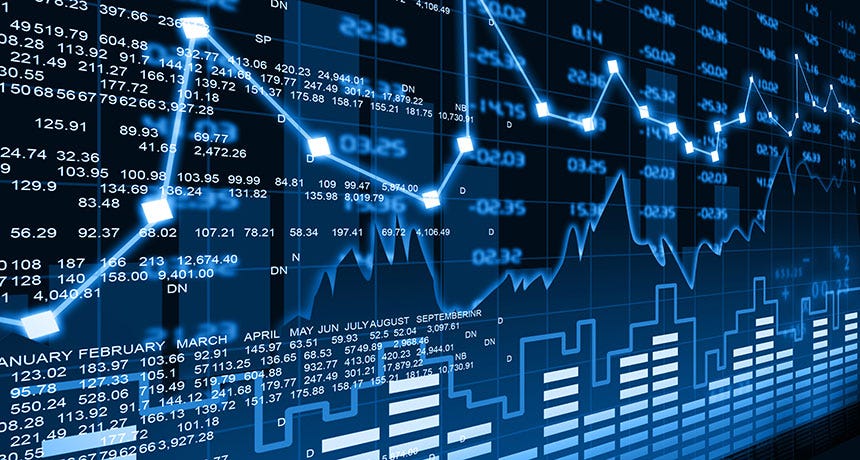
An Ultimate Guide to Becoming a Data Scientist
Becoming a data scientist is a comparatively new career trajectory that amalgamates programming knowledge, business logic, and statistics that you will get to learn during a data scientist course. Given the exponential amount of data being churned out by means of desktops, smartphones, and the huge array of IoT devices all across the globe, private and government enterprises are interested in garnering insight from their extensive data gathering procedures.
At 1st look, one may presume that data scientists and data analysts are the same – signifying there is a common 1-to-1 association between the two, but this isn’t the scenario.
While data scientists can carry out data analytics, they do so within the sphere of building and organizing predictive frameworks that usually include Toronto machine learning and deep learning protocols. Data scientists must also have a meta-level understanding of what frameworks are the most excellent fit for the data being scrutinized. Since every framework is a rough calculation of present and prospect environment, they need improvement which, in turn, depends on the mathematical expertise of the data scientists.
Even though data scientists aren’t data engineers, they should, in an ideal world, have a little acquaintance with the way databases are build, and the ways to drag data from the preferred DBMS (Database Management System) of an organization. Because of the extensive information requirements, including professional and academic training and/or experience, governmental agencies, research organizations, and companies are scrambling to discover qualified data scientists who have done data scientist course from a reputed institute, such as Lantern in Toronto.
What Does a Data Scientist Do?
Basically, a data scientist pulls out meaning from the varying kinds of data – such as semi-structured, unstructured, or structured – that flow into the enterprise. On any given day, a data scientist may be pulling out data from a database, arranging the data for an assortment of analyses, building and testing an arithmetical model or creating reports that consist of effortlessly understandable data visualizations.
There is a data science cycle that is not a set of rules as much as it is a heuristic:
- Data compilation
- Data arrangement
- EDA (Exploratory data analysis)
- Assessing and understanding EDA results
- Model building
- Model testing
- Model deployment
- Model optimization
The above is iterative, meaning a data scientist will be in “assessment mode” throughout the whole procedure.
To explore more about Toronto machine learning and data science courses, get in touch with Lantern today!